DP-100: Designing and Implementing a Data Science Solution on Azure
This course teaches you how to operate machine learning solutions at cloud scale using Azure Machine Learning. The course covers how information is prepared for a machine learning model, how the model is trained and published for use, and how to monitor the use of the model in Microsoft Azure.
This course is designed for Data Scientists with existing knowledge of Python and machine learning frameworks like Scikit-Learn, PyTorch, and Tensorflow, who want to build and operate machine learning solutions in the cloud.
This course prepares you for the certification exam DP-100: Designing and Implementing a Data Science Solution on Azure to obtain the Microsoft Certified: Azure Data Scientist Associate Certificate.
- Familiar with the basics of Azure and have experience in programming.
- Experience with the Python language as well as the Numpy, Pandas and Matplotlib libraries.
- Understanding the basics of Data Science, i.e., data preparation, storage and how models are trained using public libraries such as Scikit-Learn, PyTorch and Tensorflow.
Module 1: Design a data ingestion strategy for machine learning projects
Learn how to design a data ingestion solution for training data used in machine learning projects.
Learning objectives:
- Identify your data source and format
- Choose how to serve data to machine learning workflows
- Design a data ingestion solution
Module 2: Design a machine learning model training solution
Learn how to design a model training solution for machine learning projects.
Learning objectives:
- Identify machine learning tasks
- Choose a service to train a model
- Choose between compute options
Module 3: Design a model deployment solution
Learn how to design a model deployment solution and how the requirements of the deployed model can affect the way you train a model.
Learning objectives:
- Understand how a model will be consumed.
- Decide whether to deploy your model to a real-time or batch endpoint.
Module 4: Explore Azure Machine Learning workspace resources and assets
As a data scientist, you can use Azure Machine Learning to train and manage your machine learning models. Learn what Azure Machine Learning is, and get familiar with all its resources and assets.
Learning objectives:
- Create an Azure Machine Learning workspace.
- Identify resources and assets.
- Train models in the workspace.
Module 5: Explore developer tools for workspace interaction
Learn how you can interact with the Azure Machine Learning workspace. You can use the Azure Machine Learning studio, the Python SDK (v2), or the Azure CLI (v2).
Learning objectives:
- The Azure Machine Learning studio.
- The Python Software Development Kit (SDK).
- The Azure Command Line Interface (CLI).
Module 6: Make data available in Azure Machine Learning
Learn about how to connect to data from the Azure Machine Learning workspace. You’ll be introduced to datastores and data assets.
Learning objectives:
- Work with Uniform Resource Identifiers (URIs).
- Create and use datastores.
- Create and use data assets.
Module 7: Work with compute targets in Azure Machine Learning
Learn how to work with compute targets in Azure Machine Learning. Compute targets allow you to run your machine learning workloads. Explore how and when you can use a compute instance or compute cluster.
Learning objectives:
- Choose the appropriate compute target.
- Create and use a compute instance.
- Create and use a compute cluster.
Module 8: Work with environments in Azure Machine Learning
Learn how to use environments in Azure Machine Learning to run scripts on any compute target.
Learning objectives:
- Understand environments in Azure Machine Learning.
- Explore and use curated environments.
- Create and use custom environments.
Module 9: Find the best classification model with Automated Machine Learning
Learn how to find the best classification model with automated machine learning (AutoML). You’ll use the Python SDK (v2) to configure and run an AutoML job.
Learning objectives:
- Prepare your data to use AutoML for classification.
- Configure and run an AutoML experiment.
- Evaluate and compare models.
Module 10: Track model training in Jupyter notebooks with MLflow
Learn how to use MLflow for model tracking when experimenting in notebooks.
Learning objectives:
- Configure to use MLflow in notebooks
- Use MLflow for model tracking in notebooks
Module 11: Run a training script as a command job in Azure Machine Learning
Learn how to convert your code to a script and run it as a command job in Azure Machine Learning.
Learning objectives:
- Convert a notebook to a script.
- Test scripts in a terminal.
- Run a script as a command job.
- Use parameters in a command job.
Module 12: Track model training with MLflow in jobs
Learn how to track model training with MLflow in jobs when running scripts.
Learning objectives:
- Use MLflow when you run a script as a job.
- Review metrics, parameters, artifacts, and models from a run.
Module 13: Run pipelines in Azure Machine Learning
Learn how to create and use components to build pipeline in Azure Machine Learning. Run and schedule Azure Machine Learning pipelines to automate machine learning workflows.
Learning objectives:
- Create components.
- Build an Azure Machine Learning pipeline.
- Run an Azure Machine Learning pipeline.
Module 14: Perform hyperparameter tuning with Azure Machine Learning
Learn how to perform hyperparameter tuning with a sweep job in Azure Machine Learning.
Learning objectives:
- Define a hyperparameter search space.
- Configure hyperparameter sampling.
- Select an early-termination policy.
- Run a sweep job.
Module 15: Deploy a model to a managed online endpoint
Learn how to deploy models to a managed online endpoint for real-time inferencing.
Learning objectives:
- Use managed online endpoints.
- Deploy your MLflow model to a managed online endpoint.
- Deploy a custom model to a managed online endpoint.
- Test online endpoints.
Module 16: Deploy a model to a batch endpoint
Learn how to deploy models to a batch endpoint. When you invoke a batch endpoint, you’ll trigger a batch scoring job.
Learning objectives:
- Create a batch endpoint.
- Deploy your MLflow model to a batch endpoint.
- Deploy a custom model to a batch endpoint.
- Invoke batch endpoints.
1990,00 € (Excl. Tax)
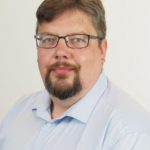
Antti “Kontti” Kontiainen
Consulting & Training
Antti is an experienced consultant and trainer who has received a lot of positive feedback about his ability to present difficult technical matters in an understandable way.
Related products
-
AZ-204: Developing Solutions for Microsoft Azure
1990,00 € (Excl. Tax)In this course you will learn how to create end-to-end solutions in Microsoft Azure. The course is tailored for developers with 1 to 2 years of practical experience in software development and basic knowledge of Azure. Students will learn how to create and manage Azure Functions and Azure Web Apps, develop solutions utilizing Azure storage,... View ArticleImplementation: Class (in Finnish), Online (in Finnish)Length: 4 daysStarting dates: Ask for details: sales@sulava.comMaterial: Microsoft English Material (MOC)Select options This product has multiple variants. The options may be chosen on the product page -
Sale!
Microsoft Sentinel SIEM One Day – How to build an effective and complete cybersecurity situation view?
790,00 €Original price was: 790,00 €.395,00 €Current price is: 395,00 €. (Excl. Tax)Learn about our cloud-based Microsoft Sentinel Security Information Event Management (SIEM) solution. This course covers how to build a comprehensive cybersecurity incident data view for your organization’s needs using a SIEM solution. This includes how to gather and organize information from a variety of sources into a real-time incident snapshot, including third-party sources such as... View ArticleImplementation: Online (in Finnish)Length: 1 dayStarting dates: Ask for details: sales@sulava.comMaterial: Sulava's english materialSelect options This product has multiple variants. The options may be chosen on the product page -
AZ-104: Microsoft Azure Administrator
1990,00 € (Excl. Tax)Does your job require managing and maintaining services in an Azure environment? The aim of this course is to prepare an IT professional towards this job role. The course consists of eleven modules. In this course you will learn the basics of Azure subscriptions and resources. We will walk you through Azure storage, virtual server,... View ArticleImplementation: Class (in Finnish), Online (in Finnish)Length: 4 daysStarting dates: Ask for details: sales@sulava.comMaterial: Microsoft English Material (MOC)Select options This product has multiple variants. The options may be chosen on the product page